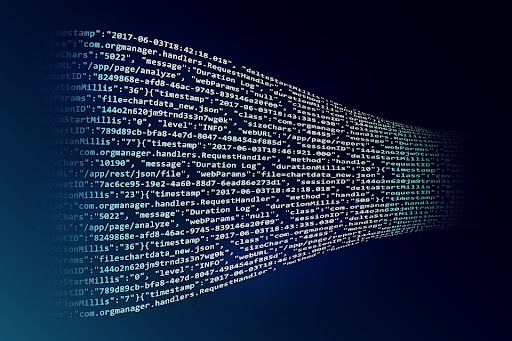
Significant data insights have made building a data lake a necessity. Data lakes are special-purpose platforms that store large volumes of raw and structured data from various sources. However, lack of attention to security has often led to serious security breaches.
If you have a data lake that trusted users could access over the internet, you need practical data lake protection tools. Without proper encryption and strict access control, you put large volumes of sensitive, financial, or customers’ data at risk. There is also the risk of ransomware; your company’s data lake becomes inaccessible until a stiff fee is paid to cyber attackers. So, your goal should be to employ the best security tools and measures.
Are you wondering where to start? This article highlights critical measures you can undertake to enhance the security of your data lake.
Encrypt Data
Data should be secured in any data management situation. But it is worth emphasizing the need for data encryption, especially sensitive information. Most data lake platforms have their data encryption methodologies. However, how you execute is also critical. No matter the data lake platform you are using, premises, or cloud, choosing a sound data encryption strategy that works with the existing infrastructure is crucial.
There is a need for efficient encryption-decryption execution. You cannot use random encryption technology and processes just for the sake of it. Select a sound encryption strategy that can protect both in-motion and at-rest states of data, especially confidential information, and other sensitive data.
Employ Machine Learning
Machine learning(ML) is not new for data management. It is especially helpful in data protection as it expedites processing and categorizing raw data, minimizing opportunities cybercriminals can exploit. Machine learning can also automate identification issues in the raw data. It can pick out suspicious files in the raw data that require security investigation. There are specific techniques of machine learning that help in data management optimization and improving data quality.
In addition, machine learning can help address issues linked to data silos. Data silos can be a severe problem and turn into overlapping monsters in the data lakes. However, ML can distribute the architecture of data models and integrate the data silo with data from other organizations to optimize operational processes to improve efficiency and data quality.
Establish Governance
A data lake is built to store all kinds of data from all sources; raw and unstructured data. However, it does not mean it has to. You should scrutinize data sources to know how the data will be processed, managed, and used. The data swamp threats are real, and avoiding them depends on many factors, such as the quality of data sources, the data coming from the sources, and the rules for data ingestion. Establishing governance makes it easier to identify details like ownership, a security protocol for sensitive data, data history, data source history, and more.
It is also essential to have role-based access, user authorization, and access authentication policies. It would be best to implement these in particular instances that provide the best data security and privacy results. Also, it is advisable to maintain the principle of the least privilege. Access is granted to some extent, just enough to perform a specific task.
Additionally, you should consider legal requirements for data protection. There are national and international laws and regulations companies have to comply with. Keeping in mind such rules, the best approach to operate efficiently is using zones within the storage layer and configuration access that are highly limited but flexible for future adjustments.
Use Partitions and Hierarchy
When data is ingested into a data lake, it is essential to store it appropriately. These data lakes are built with many standard zones that hold data based on reliability and accessibility. These zones may include:
- Temporal zone: Transient data like copies and reels streaming are kept here before deletion.
- Raw: Raw data is kept here before processing but can be further encrypted.
- Trusted zone: Data already confirmed as reliable are kept here for easy access by data analysts, scientists, and other users.
- Refined zone: Advanced data are stored here and are generally the final outputs from tools.
Therefore, you can create hierarchical zones like these to join with role-based access. This way, you reduce the probability of wrong users using or accessing sensitive data.
Implement Data Loss Prevention
To optimize the availability and integrity of data, cloud data lakes leverage persistent data in cloud storage objects. For instance, Amazon S3 is reliable in availability and secure storage. It also allows remediation of unintentional deletion or objects replacement.
You should evaluate and address all risks that could lead to data loss across all the services that store or manage data. It is critical to developing robust authorization practices that limit data deletion or update functions access. But the bottom line is to create backup and retention plans to avoid the risk of data loss.
Conclusion
Even as organizations pursue data lakes and modernization, comprehensive data security is imperative. By implementing these best practices or choosing platforms with end-to-end security built-in, you can confidently leverage the tremendous benefits of data lakes while maintaining the security of your data.